报告人:潘宝祥 副研究员,中国科学院大气物理研究所
报告时间:2025年5月20日(星期二)16:00-17:00
报告地点:西康路1号河海大学海洋学院507会议室
主持人:沈浙奇 副教授
报告人简介:
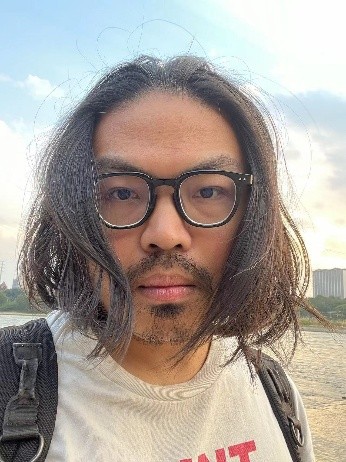
Baoxiang Pan is currently an associate research scientist at Institute of Atmospheric Physics Chinese Academy of Sciences (lAP-CAS). He obtained his PhD from University of California, lrvine. Before joining lAP, he was a research scientist at Lawrence Livermore National Lab. His research interest is to combine probabilistic machine learning with process models for better forecast across scales.
报告摘要:
Machine learning models have shown great success in predicting weather up to two weeks ahead, outperforming process-based benchmarks. However, existing approaches mostly focus on the prediction task, and do not incorporate the necessary data assimilation. Moreover, these models suffer from error accumulation in long roll-outs, limiting their applicability to seasonal predictions or climate projections. Here, we introduce Generative Assimilation and Prediction (GAP), a unified deep generative framework for assimilation and prediction of both weather and climate. By learning to quantify the probabilistic distribution of atmospheric states under observational, predictive, and external forcing constraints, GAP excels in a broad range of weather-climate related tasks, including data assimilation, seamless prediction, and climate simulation. In particular, GAP is competitive with state-of-the-art ensemble assimilation, probabilistic weather forecast and seasonal prediction, yields stable millennial simulations, and reproduces climate variability from daily to decadal time scales.